White papers
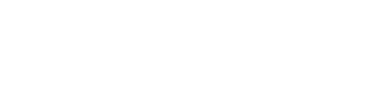
Use our white paper search or select a quick entry below to display white papers based on product type, supplier or application.
The 10 latest white papers
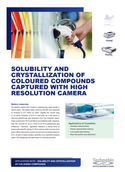
Solubility and crystallization of coloured compounds captured with high resolution cameras
Using high-resolution imaging to witness the crystallization process of coloured compounds
View white paper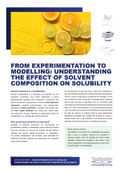
From experimentation to modelling: understanding the effect of solvent composition on solubility
Learn how to select the best solvents for solubility to optimize your crystallization process
View white paper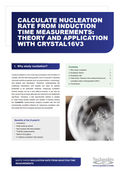
Calculate Nucleation Rate from Induction Time Measurements. Theory and Application
Discover a fast and reliable method to measure nucleation in your crystallization process
View white paperWhite papers by application
White papers by product type
Promote your white papers on chemeurope.com
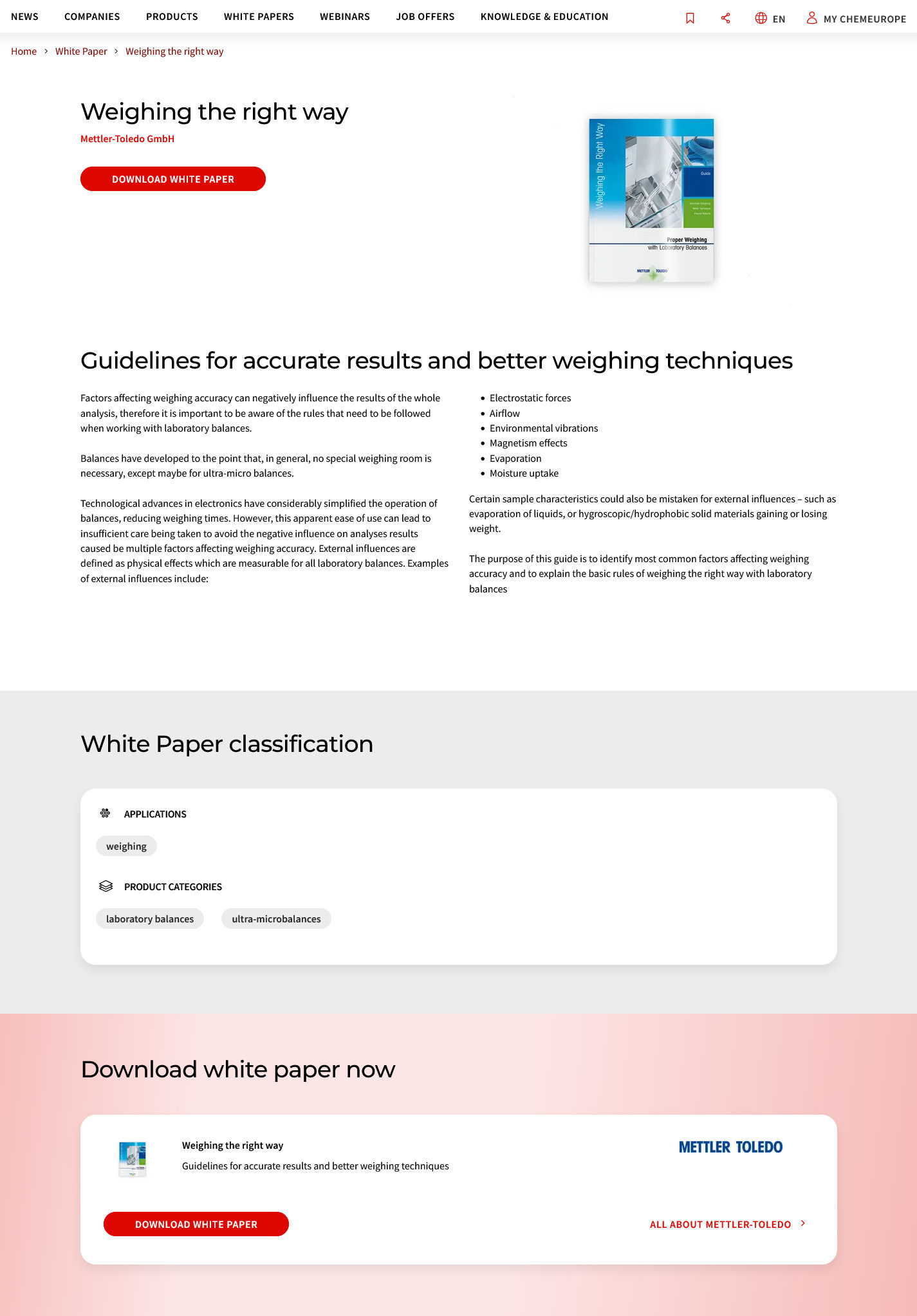
You spend a lot of time writing white papers and technical articles and offer them on your website. But the access figures are sobering. What can you do to make your white papers reach more interested parties?
On chemeurope.com, which has over 2.3 million users, your potential customers search specifically for white papers and technical articles that explain methods and explain applications.
You receive high-quality sales leads
You position your company as an expert in your field of expertise
Once written, your content generates sales leads again and again
Curious? Learn more now
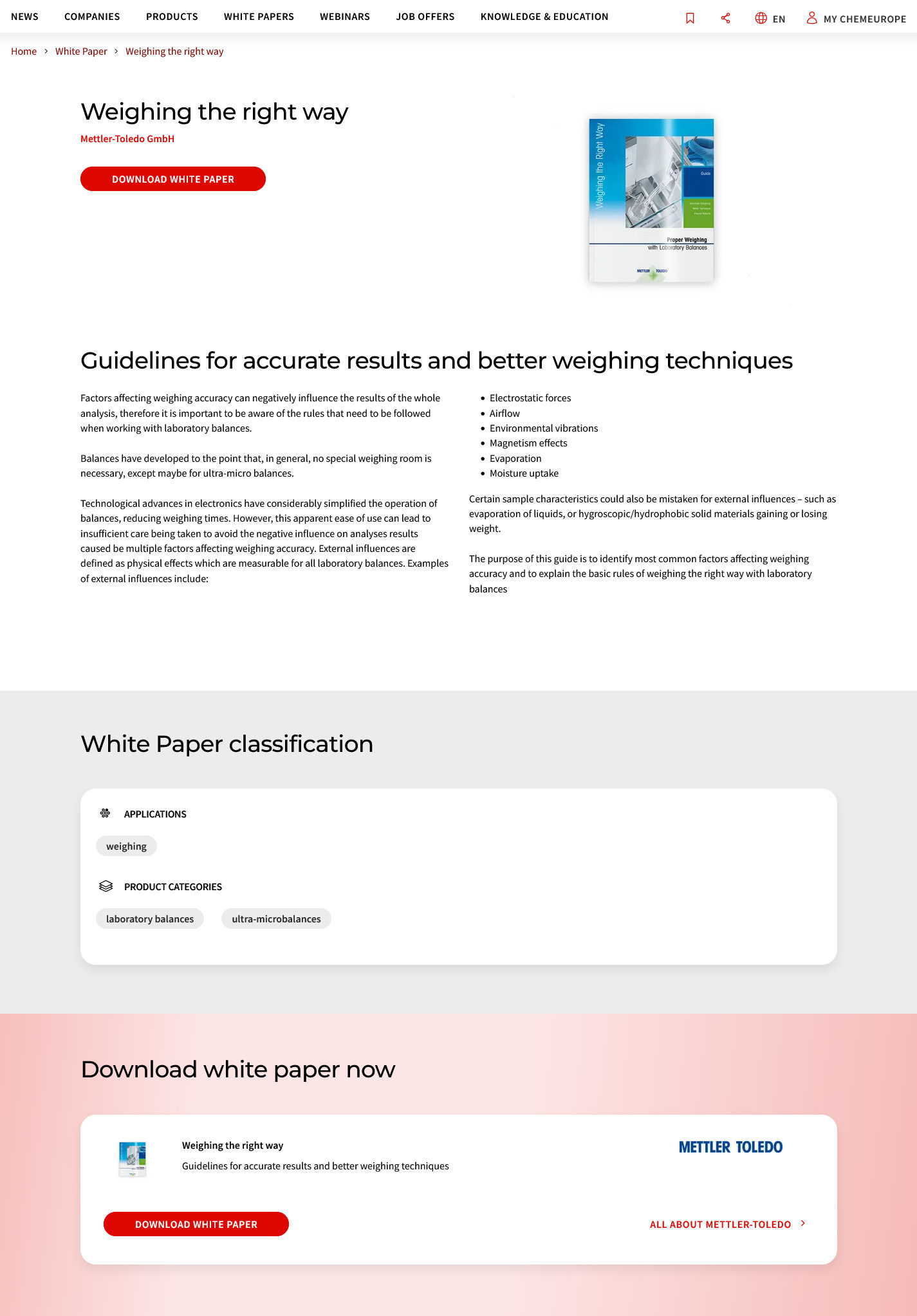